Retail Trade Area Analysis: Concepts and New Approaches
Choosing a retail site in the absence of sound trade area analysis is a lot like flying an airplane with blinders: It forces a business to commit itself to a course in the absence of vital information such as store patronage, local market opportunities, competing businesses, and barriers that would dissuade consumers from visiting the site.
Geographic Information System technology is a fundamental tool for analyzing retail trade areas today. This technology removes site selection "blinders" because it identifies and illustrates the crucial factors for site selection within a geographic framework.
Essentially, trade area analysis is a methodology, process or technique that provides a basis for understanding, visualizing and quantifying the extent and characteristics of known or approximated trade areas.
Trade area analysis provides the foundation for:
Trade area analysis also employs theoretical techniques that are used to approximate the potential patronage area. These techniques are used in cases where customer level data is not available. Three types of theoretical approaches are commonly employed in trade area analysis, including:
Radial Studies:
Radial or ring based analysis is performed by selecting and evaluating demographic variables that fall within a pre-defined distance from a store location. This technique assumes that the trade area is circular, with the store at its center. Ring analysis does not account for barriers such as rivers or railroad tracks that may cross through a trade area and restrict access to a retail site. Consequently, radial studies are a simplistic approach that can result in an incorrect delineation of the trade area and errors of omission or commission.
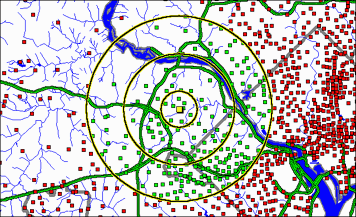
Figure 1. Location of store, showing 1, 3, and 5 mile radii. The dots indicate the locations of demographic samples. Red colored dots fall within the 5-mile radius. Note that samples located across the river would be included in the 5-mile demographic summaries for this site.
Gravity Models:
Gravity models, or spatial interaction models, define a trade area based on its attractiveness relative to other trade areas. These models provide an approximation of store trade area by putting the distribution of all locations (including competitors) into a geographical context and evaluating each location's relative attractiveness. Typically, a distance decay curve is used to model the spatial interaction of individual locations. Often size of the store, or store sales if available, is used to drive the attractiveness parameter.
Gravity models are more sophisticated than simple radial approximations, but they still do not account for logistical barriers and they are limited by the availability and accuracy of competitor data. Moreover, gravity models basically are sophisticated algorithms, which may not be appropriate for non-technical analysts.
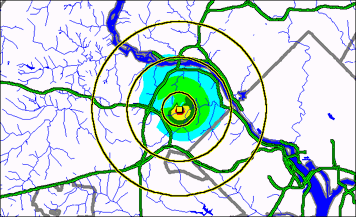
Figure 2a. Gravity based patronage probability model showing the theoretical store trade area. The blue – green – yellow – red progression represents zones of increasing patronage probability.
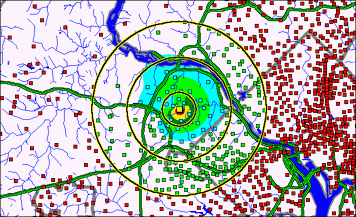
Figure 2b. Gravity based patronage probability model showing the locations of demographic sample sites. Blue colored dots fall within the patronage probability zones. Green colored dots indicate the locations of sample sites that fall within the 5-mile radius but are not within the patronage probability zones.
Drive Time Analyses:
Recently, GIS based tools for modeling the drive time or drive distance from a location have become available. These tools use digitized roadway systems that indicate the type of road such as a city street or a divided highway. Speed limits are assigned based on the type of road, the mode of transportation (car, truck, motorcycle, etc.), congestion parameters, and the time of day. These parameters are used to dictate the ease of traveling along road segments. Through this process, a polygon is generated to represent the extent to which a vehicle can travel outward from the site in all directions along the existing roadway system. Unlike the radial distance or gravity model-based trade area approximations, GIS based drive time analyses account for logistical barriers.
Drive time analyses are generally considered to be valid for “convenience” store scenarios, where patrons are expected to go to the closest or most logistically convenient location. Since this analysis is governed by the presence of properly located and attributed roadway systems, the accuracy of the drive time analysis can be limited by the availability of accurate and up-to-date digitized road data.
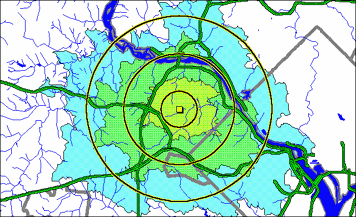
Figure 3a. Drive time analysis showing areas that can be reached within 5, 10 and 15-minute drive times.
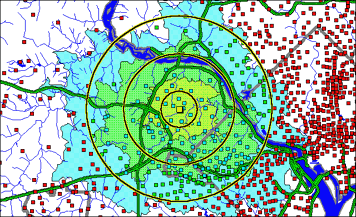
Figure 3b. Drive time analysis showing the location of demographic samples. The blue colored dots represent the demographic sample sites that fall within a 10-minute drive time. Green colored dots represent demographic sample sites that fall within the 5-mile radius, but fall outside of the 10-minute drive time polygon. Conversely, red colored dots that fall within the 15-minute drive time polygon represent demographics that would not be included using a traditional 5-mile radius approach.
Customer Level Data Drives Radial Filter Analysis Technique
The recent availability in-house customer-level, point-of-sale data is creating new ways for retail organizations to explore and evaluate the spatial characteristics of their customer base and the marketplace. Customer point-of-sale data is collected via in store surveys, “courtesy card” programs, license plate surveys, credit card transactions or through raffles or business card collections. Typical point-of-sale data contains a record of what was purchased, when it was purchased, where it was purchased and the name and address of the purchaser. This address-based data can be geocoded to provide a geographically referenced position for each customer.
Historically, analysts were forced to take address-level data and aggregate it into existing postal or census boundaries in order to summarize and map customer counts or revenue. In many cases, this level of aggregation would "smooth out" or homogenize important subtle variations in the geographic distribution and characteristics of a trade area. This is particularly troublesome in cases where the trade area is small and clustered around an area near the store, as is the case in most convenience stores.
In order to alleviate this limitation, leading GIS companies use a radial filter-based trend surface modeling application that analyzes trade areas directly from customer level point-of-sale data. This type of analysis provides a very accurate and precise measure of the spatial distribution and characteristics of store trade areas. Moreover, because customer-level point of sale data is used, the effects of logistical barriers are automatically accounted for.

Figure 4. Map showing the location of customers.
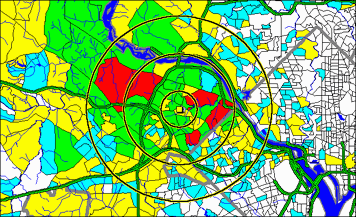
Figure 5. Color thematic trade area map showing concentration of revenue. This map was produced by summarizing the customer revenue data according to the block groups within which the customer locations fall. Colors ranging from blue – green – yellow – red represent the progression from low to high revenue.
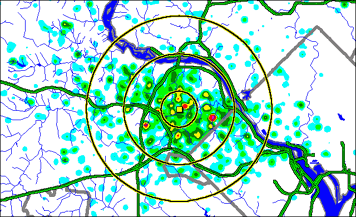
Figure 6a. Revenue based trade area map produced using radial filter based application. Colors ranging from blue – green – yellow – red represent the progression from low to high revenue. This analysis clearly shows discrete pockets of customer/revenue strength. Note how the “hotspots” identified using radial filter analysis are small and discrete, and are not constrained by pre-existing census geographic boundaries.
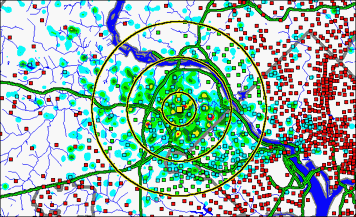
Figure 6b. Radial Filter trade area map showing the location of demographic sample sites. Note that blue colored dots represent demographic sample sites that fall within the actual store trade area. Green colored dots represent those demographic samples that fall within a 5-mile radius, but do not fall with the stores’ trade area.
Comparative Demographic Analysis:
Median | ||||||||
Population | White | Black | Asian | AmerInd | Other | Hispanic | Income | |
1 Mile | ||||||||
Total | 9,217 | 7,798 | 191 | 1,156 | 10 | 62 | 562 | $98,909 |
Percent | 100.0 | 84.6 | 2.1 | 12.5 | 0.1 | 0.7 | 6.1 | |
3 Miles | ||||||||
Total | 73,098 | 62,616 | 2,033 | 7,276 | 133 | 1,040 | 5,548 | $102,970 |
Percent | 100.0 | 85.7 | 2.8 | 10.0 | 0.2 | 1.4 | 7.6 | |
5 Miles | ||||||||
Total | 235,832 | 192,884 | 12,877 | 23,994 | 458 | 5,619 | 22,232 | $93,448 |
Percent | 100.0 | 81.8 | 5.5 | 10.2 | 0.2 | 2.4 | 9.4 | |
5 Min Drive Time | ||||||||
Total | 27,359 | 23,280 | 671 | 3,180 | 30 | 198 | 1,743 | $105,625 |
Percent | 100.0 | 85.1 | 2.5 | 11.6 | 0.1 | 0.7 | 7.8 | |
10 Min Drive Time | ||||||||
Total | 127,220 | 107,174 | 5,450 | 12,225 | 243 | 2,128 | 9,929 | $94,993 |
Percent | 100.0 | 84.2 | 4.3 | 9.6 | 0.2 | 1.7 | 7.8 | |
15 Min Drive Time | ||||||||
Total | 396,437 | 311,225 | 31,587 | 38,628 | 845 | 14,152 | 47,148 | $85,948 |
Percent | 100.0 | 78.5 | 8.0 | 9.7 | 0.2 | 3.6 | 11.9 | |
Gravity Model | ||||||||
Total | 36,386 | 31,045 | 781 | 4,124 | 49 | 287 | 2,070 | |
Percent | 100.0 | 85.3 | 2.1 | 11.3 | 0.1 | 0.8 | 5.7 | |
Radial Filter | ||||||||
Total | 225,055 | 177,201 | 23,151 | 18,497 | 512 | 5,694 | 20,624 | |
Percent | 100.0 | 78.7 | 10.3 | 8.2 | 0.2 | 2.5 | 9.2 |
The table above shows basic demographic summaries that were obtained using radial distance, drive time, gravity modeling, and radial filter analysis methods. Note the large differences between both the summary counts and the relative percentages depending on which method was used. For example, compared with the 3-mile radius, the actual trade area demographics indicate that a store is pulling from a much larger area with over three times the total population. Similarly, the median household income measured using the 3-mile radius shows income that is more than 15% higher than the trade area based income.
Similar differences are apparent when comparing the relative distribution of population by ethnicity. For example, the radial, drive time and gravity model based estimation of the percent black population is consistently lower than the 10.3% that comprises the actual trade area demographics
Trade area analyses that are based on point of sale data have some specific advantages over the three traditional methods. For example, the radial ring method assumes that a store trade area is circular and it does not account for logistical barriers or the effects of competitors. Trade areas based on drive time analysis offer a more realistic view, particularly for a convenience store scenario. However, the availability and accuracy of road networks upon which drive time analysis is based may limit drive time analysis. Drive time analysis is of limited utility when attempting to model trade areas of destination stores that draw from specific demographic groups. Gravity modeling is a more sophisticated technique that can account for the impact of competitors and is appropriate for convenience scenarios. Small differences in the gravity model parameters can have a large effect on the resulting trade area.
In comparison, the radial filter based trade area analysis generated from customer based point of sale data accurately and precisely delineates the trade area extent and characteristics. The inherent advantages of point of sale data will undoubtedly create opportunities for software vendors and hopefully, for retail businesses that employ these techniques.
Hiç yorum yok:
Yorum Gönder